Description: Polycyclic Aromatic Hydrocarbons (PAHs) are molecules of great astronomical interests, since their infrared spectrum is detected by space telescope such as the James Webb Space Telescope everywhere in space, from the very early universe to regions where stars and planets are formed (Candian, Zhen and Tielens, 2018)
To fit the astronomical PAH spectrum and learn how these molecules are created and destroyed in space, astronomers use a database containing Density Functional Theory-computed vibrational spectra in the harmonic approximation.
Because PAHs in space are highly excited, this approximation is very crude, and we should improve on it. Anharmonic DFT-based vibrational spectra obtained with generalised 2nd order perturbation theory (GVPT2) can be calculated for PAHs (e.g. Mackie et al, 2018) but the calculations become quickly very expensive as the size increases.
In this project we will use a database of calculated anharmonic vibrational spectra for small PAHs as a train set for an Artificial Neural Network to predict the spectra of larger species and compare with experimental results.
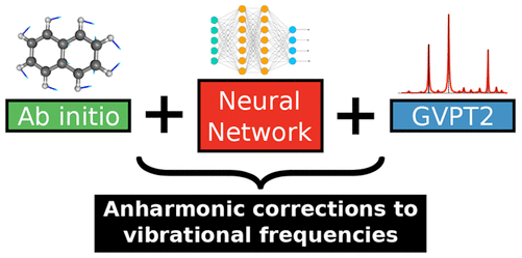
Figure 1: Image from Laurens et al. (2021), Theoretical Chemistry Accounts, 140, 66
Aim: Predict anharmonic vibrational spectra for astronomical polycyclic aromatic hydrocarbons (PAHs) using artificial neural networks.
References:
Candian, Zhen and Tielens, 2018, Physics Today 17, 11.
Mackie et al, 2018, Physical Chemistry Chemical Physics 20 (2), 1189-1197.